
.png)
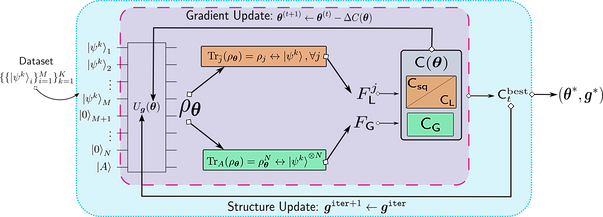
Cryptanalysis of quantum cryptographic systems generally involves finding optimal adversarial attack strategies on the underlying protocols. The core principle of modeling quantum attacks often reduces to the ability of the adversary to clone unknown quantum states and to extract thereby meaningful secret information. Explicit optimal attack strategies typically require high computational resources due to large circuit depths or, in many cases, are unknown. Here we introduce variational quantum cloning (VarQlone), a cryptanalysis algorithm based on quantum machine learning, which allows an adversary to obtain optimal approximate cloning strategies with short depth quantum circuits, trained using hybrid classical--quantum techniques. The algorithm contains operationally meaningful cost functions with theoretical guarantees, quantum circuit structure learning and gradient-descent-based optimization. Our approach enables the end-to-end discovery of hardware-efficient quantum circuits to clone specific families of quantum states, which we demonstrate in an implementation on the Rigetti Aspen quantum hardware. We connect these results to quantum cryptographic primitives and derive explicit attacks facilitated by VarQlone. We expect that quantum machine learning will serve as a resource for improving attacks on current and future quantum cryptographic protocols.